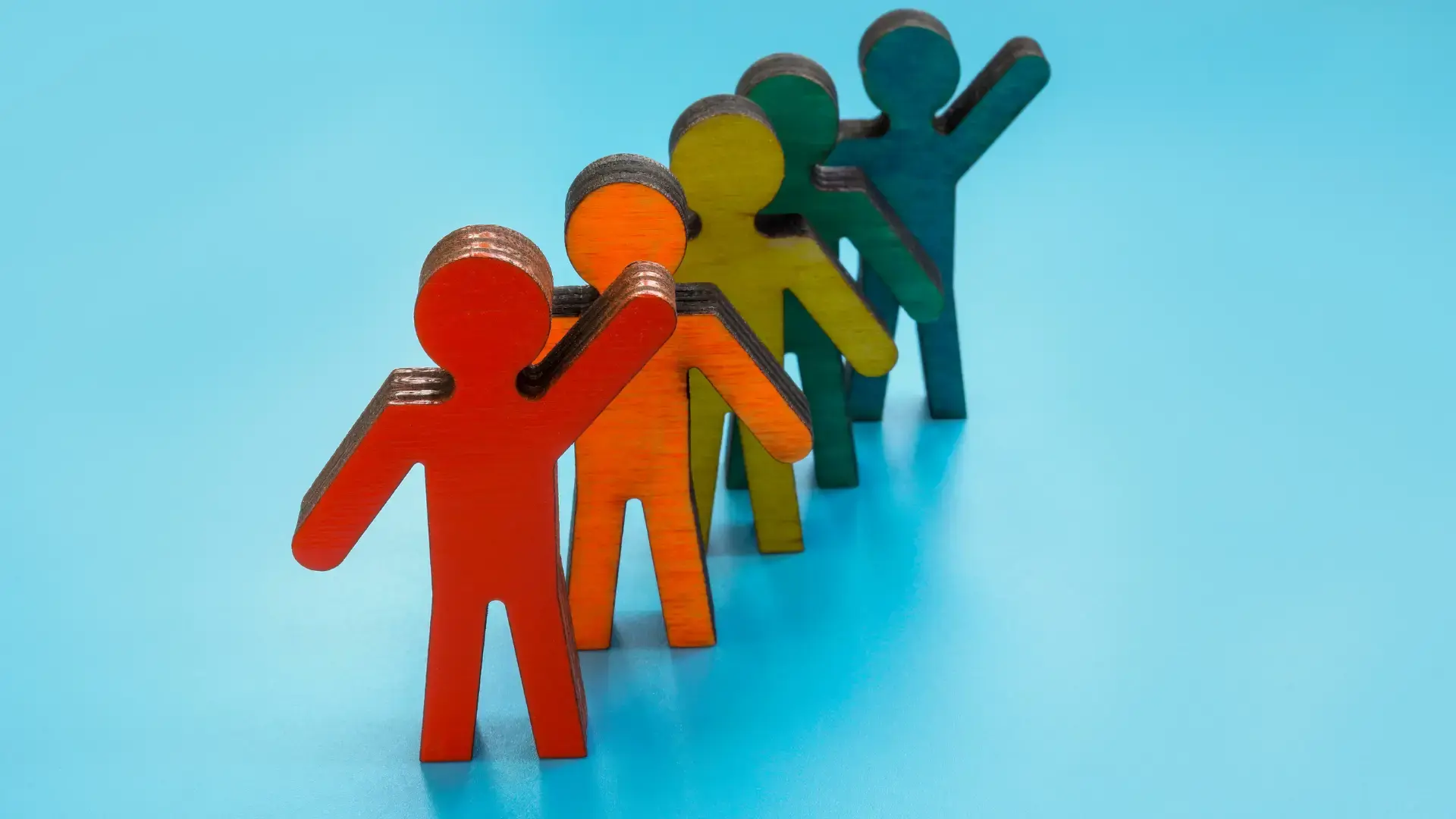
Solving the say-do gap: a privacy-first approach to robust behavioural insights
In today’s AI-driven economy, everyone from product managers and marketers to energy utilities and AI developers is chasing the same thing: behavioral insight. Not what people say they do, but what they actually do. These real-world actions power smarter products, more precise marketing, and more adaptive AI systems. Yet extracting that kind of insight without violating privacy is increasingly difficult.
In this post, we’ll explore why traditional tools like surveys often fall short, why behavioral data matters more than ever, and why collecting it ethically has become such a challenge. We’ll also look at how many current methods either overstep privacy boundaries or leave dangerous gaps, and how emerging solutions like synthetic population insights could offer a more reliable and responsible path forward.
The trouble with surveys: the say-do-gap
Surveys and interviews have long been foundational tools for understanding user behavior, but they often fail to capture the nuance and accuracy of real-world actions. The core issue lies in the type of data they collect, which is mostly attitudinal rather than behavioral. Surveys ask what people think or intend to do, not what they actually do, creating the well-documented “say-do gap.”
For example, a respondent might claim they recycle regularly, yet their day-to-day habits may not reflect that. Compounding this gap are several well-known biases. Social desirability bias can lead individuals to answer in ways they believe are socially acceptable, especially on sensitive topics. Non-response bias arises when entire demographic segments opt out, leaving results skewed and unrepresentative. Recall bias further muddies the waters, as human memory is notoriously unreliable, particularly when individuals are asked to recount behaviors from weeks or months ago. Even the phrasing of questions introduces risk, since ambiguity can result in wildly different interpretations across respondents.
These challenges contribute to the limited depth of insight that surveys can provide. Closed-ended questions may streamline data collection, but they often miss the context and complexity of behavior shaped by emotional, situational, and environmental factors. To make matters more complicated, behavioral reactivity is a real concern. The mere act of answering a survey can temporarily alter someone’s future behavior. For instance, a person asked about their eating habits might unconsciously adjust their diet in the days following the survey.
Scalability, costs, and privacy concerns of alternative methods
In light of these limitations of surveys, researchers recommend direct observation, digital analytics, and usability testing. These approaches offer a more grounded and reliable window into real behavior, free from the distortions of self-reporting and better aligned with how people act in the world. However, they are often costly, resource-intensive, and limited in scale.
Observational studies require time and trained personnel, analytics depend on digital infrastructure and consent, and usability testing captures only narrow slices of behavior in controlled settings. Despite offering deeper insights than surveys, these methods still fall short of providing a comprehensive, scalable view of human behavior, especially when privacy, representativeness, and cost are factored in.
From Fragmented insights to cohesive, privacy-friendly understanding
As traditional research methods struggle with bias, cost, and compliance, the need for new approaches has become urgent. Surveys can’t capture real behavior, observational tools are limited and expensive, and behavioral tracking raises serious privacy concerns. What’s needed is a method that is scalable, reliable, and fundamentally respectful of user privacy.
Synthetic population insights offer a way forward. Rather than collecting data from real individuals, these systems simulate population-level behavior by integrating open datasets, proprietary signals, and fine-tuned models. The result is a dynamic, privacy-safe population that reflects how real people live, move, and make decisions without exposing anyone’s identity.
This is the foundation of (Replica Italia): a high-fidelity digital twin of the Italian population. It enables organizations to test ideas, simulate responses, and explore behavioral patterns across thousands of scenarios with no real personal data and no waiting. Insights are delivered in real time, so teams can move from idea to decision faster and more responsibly.
Curious? Contact us or book a demo to see how Replica Italia can help your team move from assumptions to answers.
Tags:
blogpost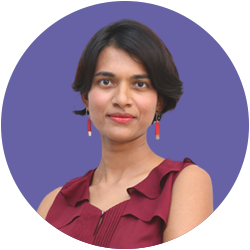